Digitalization
Innovation with AI
Our aim is to harness and translate the latest AI developments into tangible solutions that optimize our value chains and accelerate innovation. In addition to the internal research activities, BASF is strongly engaged in collaboration with various academic institutions in the field of AI.
BASF and the Technical University of Berlin have been cooperating since 2019 under the BASLEARN program to develop practical new machine learning (ML) models and mathematical algorithms to address fundamental questions in chemistry. Other academic partners in the field of machine learning include the Massachusetts Institute of Technology (MIT), Imperial College London, KU Leuven, as well as McMaster University and the University of Waterloo in Canada. In the context of our quantum computing activities, we also develop and test ML algorithms, known as "quantum machine learning."
Through a large-scale partnership between Imperial College London and BASF, advanced chemical processes have been developed to help make the chemical sector more efficient and sustainable. This research work has won the prestigious Computers & Chemical Engineering (CACE) Best Paper Award 2023. (More information)
AI is a key element of the corporate strategy, and we leverage BASF's sheer size and unique data pool, to create significate benefits. At BASF’s digital hubs worldwide, the company builds its own AI competencies, covering profiles from technical leads, cloud engineers, software/AI engineers, over data engineers and data scientists to dashboarding specialists, as well as specific or highly specialized profiles, e.g. image analysis, forecasting or natural language processing. These AI experts drive and implement the various AI initiatives across the company together with the divisions. We offer interesting work and development opportunities for domain experts with advance digital skills and dedicated AI experts who want to leverage the potential of data science, the latest technologies, and data sources to drive the digital transformation in the chemical industry.
Examples of published papers (not complete):
- Alexander Thebelt, Calvin Tsay, Robert M. Lee, Nathan Sudermann-Merx, David Walz, Behrang Shafei, and Ruth Misener. (2022) "Tree ensemble kernels for Bayesian optimization with known constraints over mixed-feature spaces.”
- Ben Tu, Axel Gandy, Nikolas Kantas, Behrang Shafei (2022) "Joint Entropy Search for Multi-Objective Bayesian Optimization"
- Jose Pablo Folch, Shiqiang Zhang, Robert M. Lee, Behrang Shafei, David Walz, Calvin Tsay, Mark van der Wilk, and Ruth Misener. (2022) "SnAKe: Bayesian Optimization with Pathwise Exploration.”
- Kasmaee, A. S., Khodadad, M., Saloot, M. A., Sherck, N., Dokas, S., Mahyar, H., & Samiee, S. (2024, December). ChemTEB: Chemical Text Embedding Benchmark, an Overview of Embedding Models Performance & Efficiency on a Specific Domain. In NeurIPS Efficient Natural Language and Speech Processing Workshop (pp. 512-531). PMLR. https://proceedings.mlr.press/v262/shiraee-kasmaee24a.html or https://arxiv.org/abs/2412.00532
-
Semnani, P., Bogojeski, M., Bley, F., Zhang, Z., Wu, Q., Kneib, T., Herrmann, J., Weisser, C., Patcas, F., & Müller, K. R. (2024). A machine learning and explainable AI framework tailored for unbalanced experimental catalyst discovery. The Journal of Physical Chemistry C, 128(50), 21349–21367. https://doi.org/10.1021/acs.jpcc.4c05332 (American Chemical Society Editors’ Choice)
-
Thielmann, A., Reuter, A., Weisser, C., Säfken, B., Kant, G., & Kumar, M. (2024). STREAM: Simplified topic retrieval, exploration, and analysis module. In Proceedings of the 62nd Annual Meeting of the Association for Computational Linguistics (ACL (Volume 2: Short Papers) (pp. 435–444). Bangkok, Thailand: ACL. https://doi.org/10.18653/v1/2024.acl-short.41
-
Thielmann, A., Weisser, C., & Säfken, B. (2024). Human in the loop: How to effectively create coherent topics by manually labelling only a few documents per class. In Proceedings of the 2024 Joint International Conference on Computational Linguistics, Language Resources and Evaluation (LREC-COLING) (pp. 8395–8405). https://aclanthology.org/2024.lrecmain.736/
-
Thielmann, A. F., Kumar, M., Weisser, C., Reuter, A., Säfken, B., & Samiee, S. (2024). Mambular: A sequential model for tabular deep learning. arXiv. https://arxiv.org/abs/2408.06291
-
Thielmann, A. F., Samiee, S. (2024). On the Efficiency of NLP-Inspired Methods for Tabular Deep Learning. NeurIPS 2024 Workshop on Efficient Natural Language and Speech Processing (ENLSP-IV) https://proceedings.mlr.press/v262/shiraee-kasmaee24a.html or https://arxiv.org/abs/2412.0053
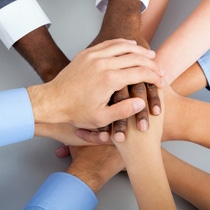